How AI is Used in Asset Management
With Artificial Intelligence (AI) entering the picture, asset managers have begun to deploy transformative impact of AI in their front, middle, and back operations. In particular, AI is transforming the workflows of analysts, data scientists and engineers, and asset managers through Natural Language Processing (NLP) techniques. Read on to find out how AI and NLP is used in asset management today.
AI Minimizes Human Error in Research
One key benefit the use of AI in asset management is the ability to minimize human error.Typically, research and business analysts in hedge funds, private equity, venture capital, and corporate finance spend hundreds of hours manually attempting to identify actionable in hundreds of unstructed text data sources, such as news, social media, blogs, filings, etc.
New data is generated at such a volume and velocity that human error in the research is inevitable. Inaccurate or outdated data can lead asset managers in making the wrong decisions and cause an asset management firm serious losses in revenue.
NLP is a subset of AI that enables computers to understand text and audio data in the same way that humans can, with the human language. Automating the analysis of text data with the right AI solution can help analysts in minimizing human error.
Role of NLP in Asset Management
When NLP is applied to unstructured text, specific words from data can be detected and the most relevant facts can be extracted which can provide valuable insights on the sentiment around an existing portfolio company, an M&A target, or identify new investment opportunities.
When combined with Automated Machine Learning (AutoML), the process of searching for specific key words and extracting relevant information can be extremely fast and accurate.
Analysts can then obtain specific data points in real-time without having to be stuck to a monitor scrolling through endless sources.

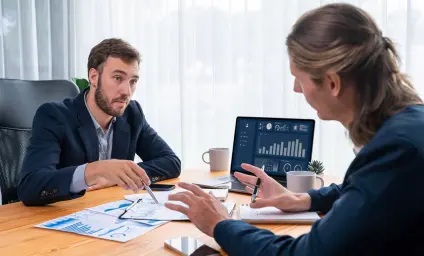
Ways AI is Used in Asset Management
Investment Research
Researching a company for investments is often difficult and time consuming. If corporations were transparent about all of their policies, actions, employee engagement and turnover, sustainable impact initiatives, and more, it would be much easier. However, the fact that corporations are not forced to disclose certain information to the public makes it difficult for investment, portfolio, and asset managers to understand and predict the long-term financial impact of a company’s actions.
By the time analysts find information using manual methods, new information is generated that could negate the previous insights.
For asset managers to identify clear next steps to take with their portfolios, they need to have a well-rounded view on the company, its products and/or services, the sentiment around the company, its interactions with competitors and consumers, and more.
Any negative or positive news, review, social post, etc can impact a company’s financial direction, especially when dealing with large corporations. For example, most corporations aren’t forced to disclose employee diversity.
However, if a corporation hasn’t disclosed that information but the truth about a lack of diversity within a company’s hiring practices is revealed, that can affect the company’s reputation and cause a negative stock price movement.
With AI and ML, analysts and data scientists can take the noise out of data mining, automate the research and extraction process, and perform sentiment and relevance analysis on social media, news, and more.
Accurate sentiment analysis is used to determine the overall sentiment from the data text and source about the company and its service or product while relevance analysis is used to determine the relevance of the news in relation to the financial team’s specific goals.
Portfolio Construction with AI / ML / NLP
With data mining and business analytics, financial teams can identify patterns and build AI models around them. Data scientists and engineers can use AI to create automated screening factors on specific events like mergers and acquisitions, interest rates, trade wars, and natural disasters. By seeing how past events have impacted a company’s portfolio, the information can be used to predict future events on a company’s stock price.
For example, by analying the impact of a historic pandemic, such as SARS, on the retail industry, it can be easy to predict the likelihood of how COVID-19 will impact the retail industry given similar circumstances. Additionally, by analyzing the impacts of previous natural disasters on specific industries, one can predict how future disasters will impact those same industries, and so on.
Another way that NLP can help with portfolio construction is to identify new investment opportunities, boost returns, and manage risk. Since corporations aren’t so transparent about many of their actual practices, NLP can find information by digging deeper than surface news.
For example, it’s nearly impossible to tell if a company is really following its ESG mandate. NLP can be used to monitor a company’s ESG compliance by searching through thousands of documents, tagging unstructured data, and revealing hidden information for analysts to review.
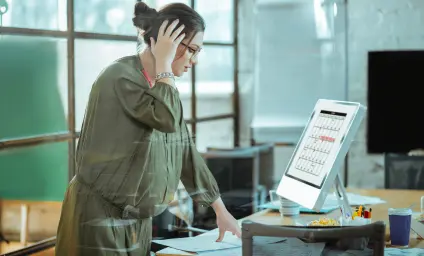
Discover Insights
Resources